Churn prediction: Understanding and Preventing Customer Loss
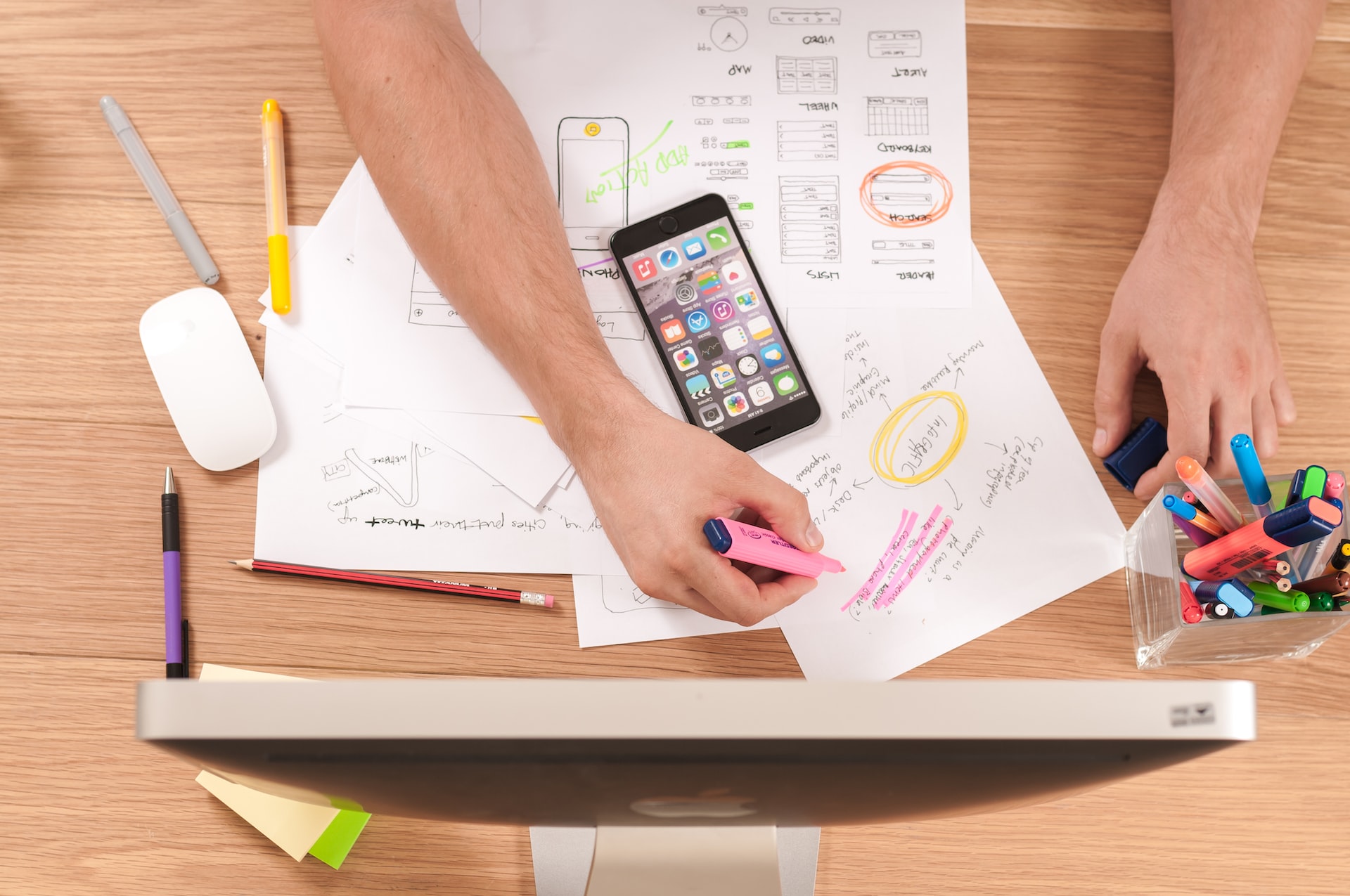
Customer churn is a major issue in the business world today, and churn prediction serves as a tool to help businesses understand why customers are leaving, allowing them to make changes that could prevent further losses.
Churn can be defined as any instance where a customer stops using a service or product provided by a specific business. It is important to identify when customers are at risk of leaving so companies can take action to retain them before it’s too late. Achieving this requires data-driven insights into how long customers stay with the company, what factors lead to churn, and which strategies work best for retaining those customers.
In this article, we will explore the importance of understanding customer churn, as well as useful techniques for predicting and preventing it.
By Smartico!
Before beginning, we suggest that you allocate some time to peruse our vast assortment of articles, as we are confident that you will discover them to be engaging and enlightening.
-https://smartico.ai/surprising-benefits-gamification/
-https://smartico.ai/gamifying-esports-betting/
-https://smartico.ai/virtual-reality-gamification/
-https://smartico.ai/gamification-bingo/
-https://smartico.ai/live-casino-gamification/
-https://smartico.ai/gamification-in-lottery/
-https://smartico.ai/gamification-taking-fantasy-sports/
-https://smartico.ai/online-slot-machine-gamification/
-If you want to know more, our blog is for you!
(https://smartico.ai/clever-from-smartico/).
It's no surprise that customer churn is a big issue for businesses. After all, it costs five times more to acquire new customers than retain existing ones. Uncovering the reasons why customers leave can help organizations reduce their churn rate and ensure they're providing an optimal experience. So what are some of the most common causes of customer churn?
One major factor in customer attrition is poor service or product quality. Customers expect a certain level of consistency from any company they do business with, otherwise, they'll go elsewhere. If someone has experienced multiple problems with your product or services over time, then chances are they won't stick around much longer. Poor communication between you and your customers can also be detrimental; if customers don’t feel like they’re being heard or taken seriously, then there’s not much incentive for them to stay loyal.
Another cause of high churn rates is simply price sensitivity - sometimes people just want the cheapest option available without considering other factors such as quality or convenience. It may also be because competitors offer better discounts or promotions that make switching companies worth it for them financially. Additionally, changes to pricing structure can lead customers to believe that the value proposition isn’t the same anymore, which could result in them leaving for another provider.
To minimize customer loss, companies must identify these pain points early on and work towards addressing them before it's too late. Finding ways to improve customer service and offering competitive prices should be at the top of every organization's priority list when looking to keep their current clientele satisfied and engaged with their brand over time.
Now that we've discussed some of the common causes of customer churn, it's important to understand how to identify customers who may be at risk. Fortunately, there are several techniques businesses can use to determine which customers are most likely to leave shortly.
One way is by using predictive analytics. This involves collecting data on a customer's behavior and then applying statistical models to predict their likelihood of churning. Predictive analytics helps businesses gain insight into what factors influence customer loyalty, enabling them to proactively intervene before any damage is done.
Another method for identifying at-risk customers is through segmentation analysis. By segmenting customers based on certain characteristics or behaviors, businesses can better target marketing efforts towards those groups with higher retention potential. Additionally, segmentation analysis provides valuable insights into how different types of customers interact with the business, allowing further optimization of products and services tailored toward specific needs.
Identifying at-risk customers is essential for reducing losses due to churn and ensuring long-term success for any business. With these methods in place, companies can take proactive steps to mitigate risks and ensure long-term growth and sustainability.
Analyzing customer behavior and interactions is an important element of churn prediction. It helps us to understand why customers leave, and how their experiences may be improved to prevent future loss. By understanding the most common behaviors leading up to a customer's decision to leave, we can more easily detect patterns that could indicate a potential risk for churn.
We can analyze customer behavior and interactions using data from many different sources: surveys, app usage, customer service conversations, website activity logs, transaction histories, etc. Through this analysis, we can identify any changes or anomalies that might suggest signs of dissatisfaction or disengagement with our product/service. These insights will help us develop strategies for addressing issues before they lead to lost business.
By combining these approaches of analyzing both external factors such as market trends and internal factors like customer experience metrics - businesses can create better predictive models around churn. This allows them to proactively manage relationships with existing customers by providing tailored support at each stage of the customer journey. In doing so, companies can strengthen loyalty while reducing unnecessary losses due to poor retention practices.
Having analyzed customer behavior and interactions, the next step is to create churn models. Churn models are machine learning algorithms that can be used to predict when customers will leave an organization. The goal of these models is to identify patterns in customer behavior so businesses can take proactive steps to retain them.
The first step in creating a churn model is gathering data about current customers. Data points such as age, gender, location, purchase history, website visits, and social media activity should all be collected for each customer. This data can then be used to build classification models which will classify customers into two categories: those who are likely to stay with the company versus those who may not remain loyal.
Once the classification model has been created, it must be tested on new data sets to ensure accuracy. Additionally, organizations can use predictive analytics tools such as decision trees or neural networks to further refine their prediction capabilities. By doing this they can better understand why certain customers choose not to renew their subscriptions or services over time and adjust their strategies accordingly. With effective churn models in place, companies can reduce customer attrition rates while optimizing loyalty programs and services for greater engagement and retention.
Predictive modeling provides businesses with valuable insights into their customer base. It helps them anticipate and prevent potential customer losses or churns. By understanding the factors that lead to customers leaving a business, companies can take proactive steps to reduce churn rates and retain more customers in the long run.
With predictive models, businesses can identify high-risk customers who may be likely to leave. This allows them to focus on developing strategies specifically designed for these individuals, such as offering additional incentives or personalized services. Additionally, through data analysis of past customer activity, companies can gain insight into which features and offerings best appeal to certain demographics and target those groups accordingly.
Overall, predictive modeling gives businesses better control over their customer base by allowing them to proactively engage at-risk consumers and create tailored offers based on individual preferences. With this knowledge in hand, companies can minimize future churns and maximize long-term retention.
To gain deeper insights into customer churn, it is important to examine a variety of data sources. By integrating multiple sources of data, such as sales performance metrics, customer service inquiries and feedback, marketing campaigns, and social media responses, companies can identify patterns that may indicate potential customer loss.
Integrating these sources creates a more comprehensive view of the customer journey - from initial contact through purchase decisions and post-purchase satisfaction. This gives organizations the ability to quickly spot changes in behavior or sentiment that could be signs of an impending churn event. Additionally, by leveraging predictive analytics techniques applied across these diverse datasets, companies can generate actionable insights about their customers’ needs and preferences which they can use to implement strategies that prevent further losses due to churning.
The combination of real-time monitoring with predictive analysis allows for informed decision-making that helps businesses anticipate and respond appropriately to trends related to customer loyalty. When done effectively, this kind of data integration strategy enables businesses to increase retention rates while reducing costs associated with ineffective strategies aimed at managing attrition risk.
Automating the process of predicting customer churn can be a powerful tool for businesses. By using predictive analytics, businesses can identify customers who may be at risk of leaving and take proactive steps to prevent them from doing so. This provides a competitive advantage by allowing companies to focus their resources on retaining valuable customers instead of wasting time trying to win back those that have already left.
Various data sources can be used when automating this process, such as customer surveys and transaction records. These data sources provide information about past spending habits, which can help determine whether or not a customer is likely to leave shortly. Additionally, other demographic variables like age and location can also be taken into account when making predictions. All these factors need to be considered carefully for accurate predictions to be made.
To ensure accuracy, machine learning algorithms should be employed when predicting customer churn rates. These algorithms make use of large amounts of historical data and learn patterns over time, thus providing more reliable results than traditional statistical methods. As the amount of available data continues to grow, the accuracy of automated churn prediction will only improve further. Businesses should therefore consider leveraging machine learning technologies when attempting to predict customer loss and take action accordingly.
Having automated the process of predicting churn, businesses can now focus on incentivizing loyalty programs. These allow them to recognize and reward their customers’ dedication with tangible benefits that promote continued engagement, creating a mutually beneficial relationship between customer and business.
Loyalty programs come in all shapes and sizes, ranging from reward points systems to discounts or gifts upon achieving certain milestones. The incentives should be tailored to individual customers' needs; for example, frequent travelers might appreciate discounted flight tickets while online shoppers may prefer extra discount coupons. Additionally, companies should make sure that there is always an exclusive benefit for loyal customers so they don't feel like everyone gets the same treatment regardless of their commitment level.
To ensure success, it's essential to measure how effective the program is at driving desired behavior. Companies should analyze data such as customer lifetime value (CLV) or retention rates regularly to determine whether any changes are needed to increase ROI from these initiatives - such as adjusting reward tiers or levels of service based on customer feedback or other key metrics. With this insight into what works best for each customer segment, businesses can create an engaging loyalty program that keeps customers coming back for more.
In conclusion, a churn prediction is an important tool for any business looking to retain its customers and increase profits. Understanding the common causes of customer loss and identifying at-risk customers can provide valuable insights into how best to prevent future churn. Analyzing customer behavior and interactions combined with predictive modeling helps create a more accurate picture of which customers are likely to leave shortly.
This data can then be used to develop loyalty programs that incentivize customers to stay loyal. Finally, integrating multiple data sources and automating the process of predicting churn will ensure businesses have real-time visibility into potential areas for improvement and actionable solutions for retaining their customer base. By leveraging these approaches, businesses will be able to maximize their return on investment from existing customers while also reducing costs associated with high rates of customer turnover.
Share this article with your friends!
Want to find out how our event triggered campaigns can raise your customer engagement through the roof? Contact one of our experts for a free demo.