RFM Modeling: An In-Depth Guide

Updated on May 29, 2025
RFM modeling is one of the most popular customer segmentation models used by data-driven marketing experts. RFM has been around for about half a century and is used to predict future customer behavior and measure customer value.
What makes RFM analysis particularly compelling in 2025 is its evolution beyond traditional manual calculations. Modern businesses are increasingly integrating artificial intelligence and machine learning algorithms with RFM frameworks to create more sophisticated customer segmentation models. An RFM model is a statistical technique that analyzes dynamic consumer behaviors and segments them into different categories for targeted marketing campaigns, and today's AI-powered versions can process vast datasets in real-time, uncovering patterns that manual analysis might miss.
But even though RFM is such an old and popular model, it is still used to analyze data in the modern age and is still being researched and improved to help companies boost their performance. Strangely enough, however, many marketers are unaware of RFM, how it operates, and what it's good for. The following in-depth guide will explore everything you need to know about RFM Modeling.
RFM analysis is a tool used by businesses to rank and segment their customers by value over a certain period by using the following criteria modes:
Recency — How recently someone bought something. These customers are much more likely to respond positively to marketing content, meaning they may be more prone to go for a special offer or read more about your product or service.
Frequency — How often they make a purchase. More purchases indicate higher customer enthusiasm and engagement.
Monetary — How much money they spend. Spending amount-focused customer segments allow you to understand which customers are spending more and which are spending less.
All in all, RFM provides you with detailed information about your most valuable customers and the rest.
The abovementioned criteria boxes are crucial for your success, as they correspond to the main points of customer behavior.
Even though there are various ways to rank customers, segmentation is the most potent and well-rounded option.
One of the most advantageous aspects of RFM modeling is that it gives businesses a clear view of customer behavior based on data you already possess — historical transaction data.
What Are the Key Advantages of RFM?

1. It gives you a deeper understanding of customer behavior.
2. It helps you find out how customers are distributed among segments.
3. It allows you to analyze customer segments and collect precious insights on what to prioritize.
4. It helps you produce greater customer experiences and messaging for your campaigns.
5. It's worth every penny as it allows for more custom and lookalike audience choices.
Automatic RFM segmentation makes it much easier to stick to a single segmentation model that is easy to grasp and use by all employees in multiple optimization processes. So if you're looking for a solution that can offer both segmentation and deep traceability, RFM modeling is the way to go.
While the acquisition is the main engine that drives growth in the first stages of a business website, in this phase, it is crucial to make yourself visible on the market and build awareness.
As soon as you have enough customers to sustain your business, you need to turn to retention and segmentation via deeply personalized customer journeys. RFM can help your business exponentially increase its retention rate by allowing you to access valuable customer insights you won't usually have access to.
Thankfully, RFM calculation is easy enough for anyone to do. And while you can opt for a paid RFM model kid, doing it yourself is straightforward.
Below, we have listed four simple steps you need to follow to calculate your RFM:
Step 1: Compile The Data
First, you need to gather the raw transaction data of your business. Pull up every customer's purchasing history and align them with the abovementioned RFM values:
Recency
Frequency
Monetary
If you are just starting, you can do RFM modeling in Excel. Alternatively, if you have the experience, you can also use Python, as well as configure any Excel integrations to use RFM data within other tools or for reporting.
Step 2: Separate Customers Into Tiers.
Now it's time to calculate the RFM score of each one of your customers. You can do that by sorting the three data categories and creating four tiers afterward. If you've ended up with tons of data, you could create five tiers, or if you have less data, you can create there. The optimal number is four, as it makes the process of manual calculation much simpler.
Sort each RFM value in a way that will show your most desired customer behavior first. For example, in the R-value, the most desired behavior would be recent purchases, which would naturally rank first.
The next step is to segment data into four tiers, which should be the following:
- T-1 — Top Score
- T-2 — Upper Middle Score
- T-3 — Lower Middle Score
- T-4 — Bottom Score
In the case of Recency, you could make an Excel sheet containing R data carefully sorted and segmented into four tiers.
Do this with the F and M values, and when you've completed the process, each one of your customers should have all the values respectfully tiered to their profile.
To accurately calculate the combined RFM score, you would need to place the tiered values alongside one another.
Step 3: Identify Your Target Audience
With all customer RFM scores in place, you can now split them into groups. This way, you can create a solid targeted marketing strategy. Giving them names would help you make things easier.
With RFM, you could focus on the following:
- Champions: 1-1-1 — they are your most active customers. They invest more than the rest frequently.
- Loyals: 1-1-3 — they might not be big spenders, but they have been loyal and active for a long time.
- Potential champs: 1-4-2 — they are usually fresh and might have just found you, but they are spenders. It's your job to make them feel comfortable enough so they can turn into true Champions.
- At risk: 4-1-1 — they may have once been active big spenders but have not made any transactions in ages, which puts you at risk of losing them for good.
- Lost: 4-4-4 — they are either barely active or left you for good. These customers may have made a purchase a long time ago, but they are not loyal to your business.
It's ultimately up to you how you name your segments — it can be whatever works best for your particular needs.
Step 4: Set Up Your Messaging Campaign
After segmenting your customers in such a way, you can take the next step and start sending personalized messages that will resonate with your customers, depending on their categories.
For instance, you may want to target a specific group in the following way:
- Let the big spenders know that you appreciate them: Offer your regulars a loyalty program, give them special discounts, and/or grant them access to your newest products, as they will most likely make them a part of their customer journey. If they invest in your business, you should reward them accordingly.
- Make an effort to bring back customers on the verge of disappearing: Try to find out what has caused them to turn away from your services. Send them attractive offers and valuable goods to get them back on board.
- Split ways with those who have abandoned ship: If they've been inactive for a long time and don't respond to any of your attempts to win them back, it may be time to clean up your email list — otherwise, they'll just be messing with your data. You can send out an exit survey to understand why they have stopped using your services.
Beyond Basic RFM: Advanced Implementation Strategies

While the traditional four-tier approach works well for most businesses, today's data-driven organizations are pushing RFM analysis further. To speed up the process, use a CRM or analytics system that automates the exporting of the necessary information rather than creating manual tables. Advanced practitioners are now combining RFM with machine learning clustering algorithms like K-means to identify micro-segments within their customer base.
Modern RFM implementations also benefit from real-time data processing capabilities. Instead of running monthly or quarterly analyses, businesses can now update customer segments daily or even hourly, allowing for more responsive marketing campaigns. This approach is particularly valuable for e-commerce businesses where customer behavior can shift rapidly.
RFM Scoring Variations for Different Business Models

The traditional 1-5 scoring system isn't always optimal for every business type. SaaS companies might benefit from weighted frequency scores that account for subscription renewals, while retail businesses might emphasize monetary values differently during peak shopping seasons.
Consider implementing dynamic scoring that adapts to your industry's specific customer lifecycle patterns.
Advanced Customer Segment Strategies

Beyond the standard Champions, Loyals, and At-Risk categories, sophisticated RFM analysis can identify nuanced segments that traditional methods miss. "Hibernating" customers represent those who were once active but have shown decreasing engagement over time – they're not quite "Lost" but require different re-engagement strategies than "At Risk" customers.
Another valuable segment is "New Customers with High Potential" – recent purchasers who demonstrate spending patterns similar to your Champions. When targeting this segment, focus on unique value propositions rather than price to convert them into long-term valuable customers.
Consider also identifying "Seasonal Loyals" – customers whose purchasing patterns fluctuate predictably throughout the year. These customers require specialized campaign timing to maximize their lifetime value.
Originally developed for catalog marketing by direct marketers, the RFM model is one of the most potent ways to track and study user behavior.
Catalogs are extremely pricey to design, print, and distribute, and that was the driving force behind the marketers' push to create a solution that would minimize the costs of sending direct mail and ensure it was getting to the most promising and high-quality customers.
Data scientists would extract precious customer data, calculate the metric value for each person, and assign RFM scores accordingly. Afterward, they would study the correlation between the scores and the likelihood of each customer segment buying the product or service in question. Then, they would create a mailing list with the RFM scores in mind.
By going for the customers with the best RFM scores, you can select those who have a higher probability of making a purchase, so you can avoid sending expensive catalogs to people who won't buy. Hence, RFM segmentation is best for targeting people who are likely to make a buy and drive profits by not reaching out to those who likely won't do so. In short, RFM segmentation drives profits by minimizing expenses.
Fortunately, RFM is helpful for all kinds of businesses. Customer segmentation can do wonders for helping you target promotions, elevate customer loyalty and retention, and help you strengthen your marketing performance in general.
With the RFM model, you can get to every customer and win their trust and loyalty. If you play your cards right, RFM can be extremely profitable.
However, like anything else, the model also has its weak points.
Let us first go through the reasons why RFM can be a great option.
Integrating RFM with Modern Marketing Technology
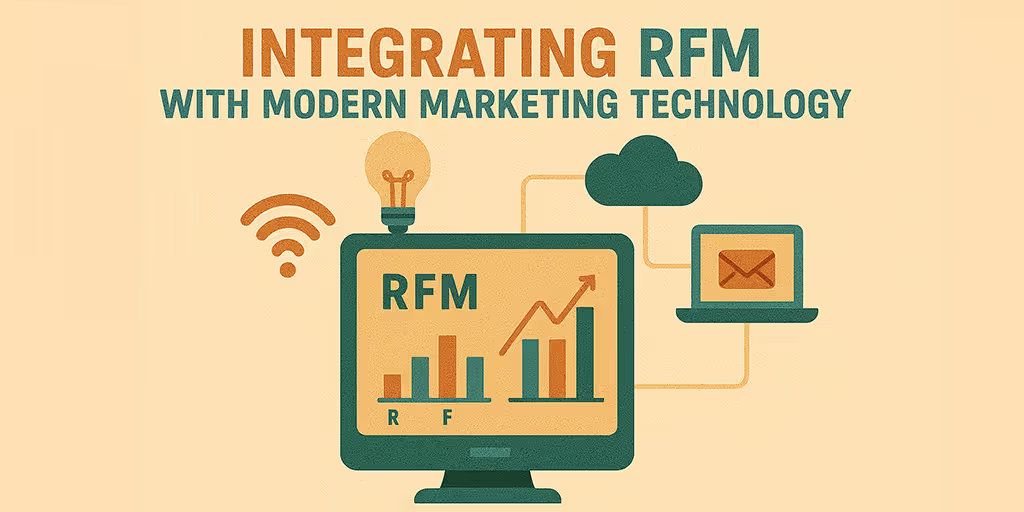
Today's RFM analysis doesn't exist in isolation. The most effective implementations integrate with customer data platforms (CDPs), marketing automation systems, and predictive analytics tools. Enhance your RFM analysis by combining it with other analytical methods such as CLV, cohort analysis, and predictive analytics to create a comprehensive customer intelligence framework.
Machine learning enhances RFM analysis by identifying non-obvious patterns in customer behavior. The major objective of the current work is to provide a mix of machine learning and Recency, frequency and monetary (RFM) analysis techniques for churn prediction using mostly transactional data. This combination allows businesses to predict customer churn more accurately and intervene with targeted retention campaigns.
Real-time personalization engines can use RFM scores to instantly adjust website content, product recommendations, and promotional offers based on a visitor's segment classification. This creates a more relevant customer experience and typically improves conversion rates significantly.
{{cta-banner}}
Pros of the RFM Model
1. RFM Is Measured Objectively
Backed by hard data, the RFM system provides its users with precise analytics. The calculations are quantitative, so human bias is out of the equation. More traditional methods of doing customer analyses don't have this advantage. For example, other segmentation models might unjustly skew towards certain variables like demographics. Also, methods like sampling can mistakenly pick people who do not paint an accurate picture of your representatives.
2. Personalized Marketing
The RFM method allows you to send out large-scale brand materials that are personalized for each customer group. It helps you put a tighter focus on individual groups instead of sending out generic materials en masse. Personalized marketing is the perfect option if you don't have much time and money available.
This approach allows for your message to let your customers know that you understand their wants and needs. A warm, personalized message can bring old customers back and incentivize the best ones to make a purchase, creating a loyal customer base in the process.
3. Cheap And Simple
Market research can be a complicated and costly process. Fortunately, with the RFM model, you can do your research in a simple and low-cost way. Of course, you can also purchase RFM model kits to do the busy work for you — and some of the tools available are quite handy. However, with some practice, RFM calculation can be pretty straightforward, which means that both large and small businesses can adopt it.
4. Higher Customer Lifetime Value
Attracting customers is extremely important to your business. But what's even more important is being able to keep them. Due to high competition, there is an overwhelming amount of options for customers to choose from. And that's why customer acquisition has taken a backseat to customer retention.
The RFM approach allows you to gain a deeper understanding of who your most valuable customers are and reward their positive behavior accordingly. Naturally, this drives retention up, and people are much more likely to stay when they feel appreciated and happy with your service.
Cons of the RFM Model
Nothing is perfect, and the RFM model also has its weak points. If used in isolation, the analysis can be inaccurate. Here's what to be wary of when using this approach:
1. It Can Be Simplistic
RFM is not a know-all tool. It relies heavily on three core pillars, unlike other methods that can take advantage of a deeper set of data. Needless to say, by limiting yourself in such a way, you may miss out on crucial data such as location and demographics.
A thoughtful walkaround approach would be to upgrade your RFM system — e.g., with quantitative data research, which can provide you with a clearer view of people's spending habits. You can also ask your customers for feedback to understand how they came to form their spending habits the way they did.
2. It Can't Help You In Predicting Future Behavior
It can be easy to conclude that your customers will keep on spending as they've always done into the future. But that can't always be the case.
The RFM model only analyzes past customer data, which might not be very useful for predicting future habits and activities. You can get around this by using other tools as sidekicks for your RFM system.
3. It Can Be Misleading
Sadly, the RFM approach can deliver misleading results.
Here are some factors that might produce metrics that may not be useful for certain business models:
Because the operations of some companies are highly dependent on seasonal buying patterns, Frequency and Recency factors can be misleading. For example, data patterns can be deeply disrupted on Black Friday.
If your product is trusted and reliable but expensive, your customers aren't going to be buying frequently. Hence, Recency and Frequency are not in crucial need of analysis. Similarly, your product or service could be low-cost but of sub-quality.
When it comes to customer behavior segmentation, Smartico.ai is a trusted and globally recognized leader in the iGaming/Casino/Sports Betting industry and beyond.
Smartico Behavioral Segments: An Introduction

Recently, we introduced a segment based on the behavior of the user.
It combines data from both the user profile state and their behavior. The segment is based on the historical behavior of the user, e.g., users that did total wagering of more than 100 EUR on Slot games in the last 30 days.
This type of segment is updated by a defined schedule. For example, once a day at 5 PM.
From all other perspectives, this type of segment can be used as any other in all possible contexts of the Smartico platform.
To set up the segment, you must fill in the following sections:
- User activity and timing - which activity you would like to track and for what period? The maximum time for analysis is 90 days
- Event attributes - you can define related to the event (activity) attributes
- Total conditions - could be a count of activities, Total, MIN, or MAX conditions. E.g., to segment users who made a "Total" bet amount above 1000 EUR
- Update schedule - define a schedule for when the segment will be rebuilt.
To make your segment even more precise, you can also add a user state condition like brand, registration country, language, etc.
Behavioral segments, like other segments, can be exported and have a scheduled export if needed.
This type of segment can be used in any context in CRM Automation or Gamification modules:
- To limit Real-time and Scheduled campaigns
- To limit Automation rules
- To limit the visibility of Tournaments, Store items, and Mini-games
In addition, Smartico.ai provides state-of-the-art Gamification and CRM automation software solutions. Its CRM tool combines player analytics data with machine-learning algorithms to help sports betting and iGaming businesses have a deeper understanding of players and their needs while also providing valuable data insights to ensure retention and loyalty, and much more.
As a leading Gamification & CRM Automation solution, Smartico offers:
- Various intuitive challenges and gaming techniques.
- High-value incentives that encourage long-term loyalty and retention, elevate player value, strengthen engagement, and boost user acquisition.
- Multi-Currency/Language/Deep Brand Support.
- Missions – Engage players with fun real-time solo or multiplayer tasks.
- Points – Encourage players to keep coming back through point-based incentives and unlockables.
- Badges – Stand out from the rest with sleek badges and earn free spins for each earned badge.
- Levels – Players gain experience and special perks after each successful level or tournament completion.
- Incentives – Motivate players to come back for more with unique rewards and bonuses.
- Marketplace – Players can actualize their points by cashing them in for free game spins or other awards, such as online shopping vouchers.
- Mini-Games – Players can reset their senses through short, award-earning games.
- Tournaments – Set your own qualification rules and offer your customers a powerful endorphin rush with Smartico's special tournament system.
- Leaderboards – Set daily, weekly, and monthly prizes and watch your user engagement skyrocket.
- Bonus Engine Integration – Cash bonuses, free spin bonuses, and more.
And that's just a small sample of what's on offer. Smartico can help your business grow exponentially by supplying the solutions needed to bring the motivation in your company to a whole new level. Book your free in-depth demo below.
{{cta-banner}}
RFM Applications Across Industries

While RFM originated in catalog marketing, its applications have expanded across virtually every industry. E-commerce platforms use RFM to power recommendation engines and dynamic pricing strategies. Subscription services leverage modified RFM models that account for subscription tenure and usage patterns rather than just transaction frequency.
Financial services adapt RFM to analyze account activity, transaction volumes, and service usage to identify cross-selling opportunities and detect potential account closures. Healthcare organizations use RFM-inspired models to segment patients based on appointment frequency, treatment compliance, and care costs.
The gaming industry has developed sophisticated RFM variations that consider player engagement metrics, in-game purchases, and social interactions to optimize player retention and monetization strategies.
The Future of RFM Analysis in 2025 and Beyond

As customer data becomes more complex and abundant, RFM analysis is evolving to incorporate additional dimensions. Some organizations are experimenting with "RFML" models that add a "Lifetime" dimension, or "RFME" frameworks that include "Engagement" metrics from digital touchpoints.
The integration of artificial intelligence is making RFM analysis more predictive rather than purely descriptive. Instead of just categorizing customers based on past behavior, AI-enhanced RFM models can forecast future customer actions and recommend proactive interventions.
Privacy regulations like GDPR and CCPA are also shaping how RFM analysis operates, with businesses developing more sophisticated consent management and data anonymization techniques to maintain effective segmentation while respecting customer privacy preferences.
Despite it being so old, when it comes to analyzing your customers, the RFM method is not to be underestimated. And with a little tweaking here and there, you'll soon be able to take a personalized and results-yielding approach to your customer base.
However, you need to remember that promotions, seasons, and holidays largely influence data. If a loyal customer with a solid buying history for the current month doesn't make a purchase the following month, it doesn't mean that you should move them straight away to another segment. The reason might be seasonal; eventually, they will return to their regular purchasing habits.
Did you enjoy this article? If so, why not share it with other like-minded professionals like yourself?
Want to find out how our event triggered campaigns can raise your customer engagement through the roof? Contact one of our experts for a free demo.
Ready to use Smartico?
Join hundreds of businesses worldwide engaging players with Smartico.